By Garret DeReus, January 7, 2024
Recent technological developments have led to the increasing use of Large Language Models (LLMs) in healthcare settings. While these tools can provide valuable support for healthcare providers, their limitations create serious risks when providers rely too heavily on them instead of exercising proper clinical judgment. Understanding how these systems work—and fail—is crucial for both medical professionals and patients.
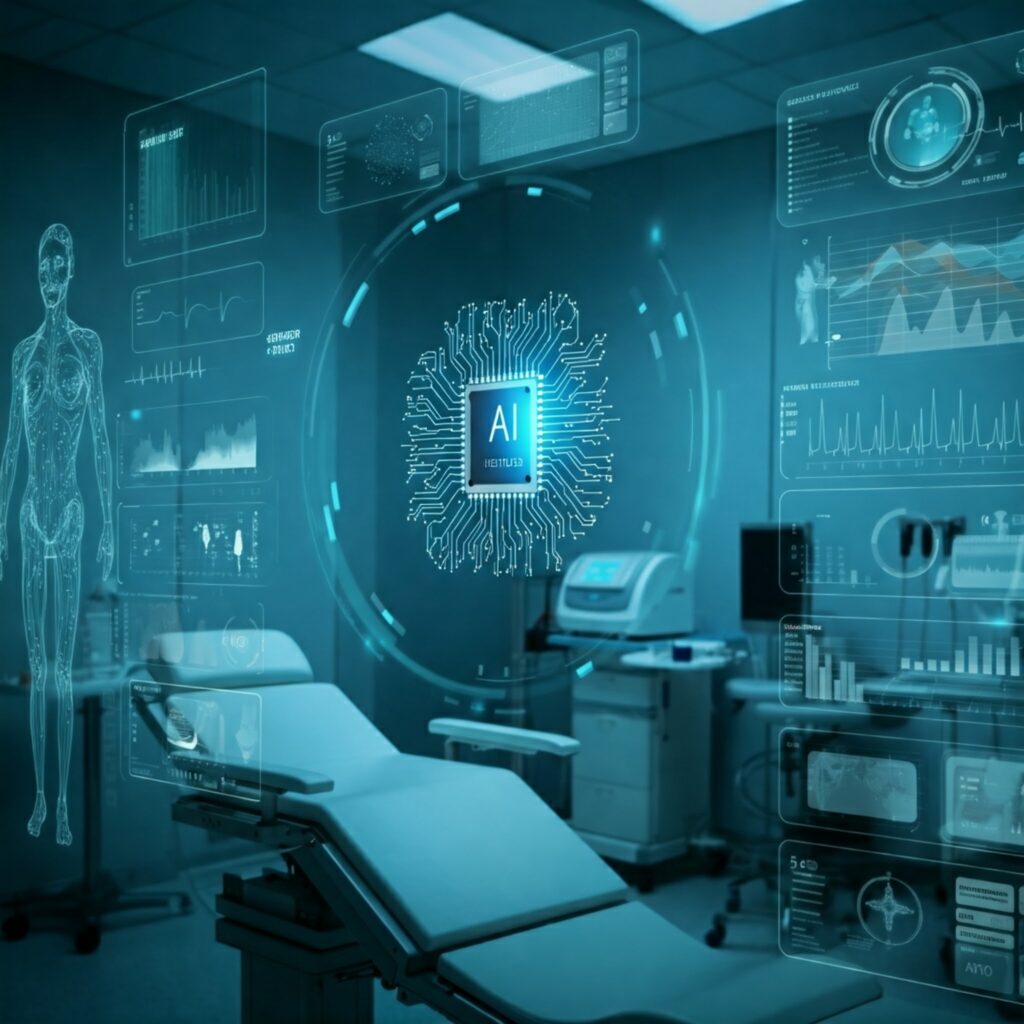
Understanding Large Language Models
Large Language Models are sophisticated tools that process and generate text based on extensive training data, much like an incredibly advanced predictive text system. These models analyze vast amounts of text – including medical literature, clinical guidelines, and healthcare documentation – to identify patterns and relationships between words and concepts. When a healthcare provider inputs a question or description of symptoms, the LLM doesn’t access a structured database of medical knowledge. Instead, it generates responses by predicting what text should come next based on patterns it observed during training. Think of it like an extremely sophisticated autocomplete function: when a healthcare provider types “patient presents with chest pain and shortness of breath,” the LLM draws upon its training to predict what information or recommendations typically follow those symptoms in medical texts.
Critical Limitations in Medical Settings
While LLMs can produce impressively detailed medical text, they fundamentally lack true medical understanding or reasoning ability. These systems don’t actually comprehend medical concepts or possess clinical judgment – they simply recognize patterns in text. This limitation becomes dangerous when healthcare providers mistake the LLM’s fluent responses for genuine medical expertise. For example, an LLM might generate a seemingly reasonable response about chest pain that matches common patterns in its training data, but completely miss subtle indicators of an uncommon but life-threatening condition that a human doctor would recognize. The system cannot truly understand the complex interplay between a patient’s symptoms, medical history, and current condition. It cannot apply medical reasoning to determine when a case requires deviation from standard protocols, and it cannot recognize when its pattern-matching approach is inappropriate for a unique patient situation. When healthcare providers rely on these pattern-matching responses instead of applying their medical training and clinical judgment, they risk missing crucial diagnostic clues that don’t fit typical patterns, potentially leading to catastrophic outcomes for patients.
Known Issues with Medical Information Accuracy
Large Language Models face significant limitations when processing medical information, creating serious risks for patient care. Unlike human healthcare providers, these systems cannot physically examine patients, assess subtle clinical signs, or integrate complex medical histories with current symptoms. LLMs generate responses based on pattern recognition from their training data, which may be outdated or contain inaccurate medical information. For example, an LLM might miss the significance of seemingly unrelated symptoms that an experienced physician would recognize as a rare but serious condition. These systems can also produce “hallucinations” – confidently stated but entirely incorrect medical information – or fail to recognize when multiple symptoms indicate a medical emergency requiring immediate intervention. Perhaps most dangerously, at least as of this article in January of 2025, LLMs cannot distinguish between typical and atypical presentations of diseases, potentially leading to missed diagnoses in cases where symptoms don’t perfectly match textbook descriptions. While these tools can be valuable for supporting healthcare providers in research and documentation, relying on them as primary diagnostic tools is a recipe for a healthcare profession to puts patients at serious risk.
The Problem of Outdated Medical Information
Healthcare providers who rely on Large Language Models also do so at the risk of relying on outdated information. LLM systems are frozen in time, only containing medical knowledge up to their last training date. For instance, an LLM trained on data through 2023 would be unaware of new treatment protocols, recently discovered drug interactions, or emerging public health threats that emerged afterward. This temporal limitation becomes particularly dangerous in rapidly evolving medical situations, such as during disease outbreaks or when new research reveals previously unknown complications of common medications. Consider a healthcare provider in 2025 consulting an LLM about best practices for treating a specific condition – the system might suggest an outdated protocol that has since been proven ineffective or even harmful. Similarly, the system would be unaware of newly identified side effects, updated diagnostic criteria, or revolutionary treatment options that became standard practice after its training cutoff. If healthcare providers blindly trust these outdated recommendations instead of independently reviewing the current medical literature and continuing education, they may inadvertently provide substandard care that fails to meet Louisiana’s medical standard of care requirements.
Legal Implications for Louisiana Healthcare Providers
In Louisiana, healthcare providers have a legal obligation to provide care that meets established professional standards. When providers rely primarily on LLMs instead of their medical training and judgment, they risk falling below this standard of care. If a patient suffers harm due to a missed diagnosis or delayed treatment because a healthcare provider followed an LLM’s recommendations without proper clinical verification, this could constitute medical malpractice under Louisiana law.
Moving Forward
Healthcare providers’ reliance on so-called artificial intelligence tools like Large Language Models will likely continue to increase in the coming years. While these technologies offer benefits when used appropriately, they should never replace proper medical assessment and clinical judgment. If you believe your healthcare has been compromised by a provider’s over-reliance on AI tools, documenting your experiences and seeking legal guidance promptly can help protect both your rights and the quality of healthcare in our community. These cases not only affect individual patients but also help establish important precedents about the appropriate use of AI in medical settings.
Louisiana law provides clear protections for patients who have been harmed by medical negligence, including cases involving improper use of technology. Understanding these rights—and acting on them when necessary—helps ensure that healthcare providers maintain appropriate standards of care as they integrate new technologies into their practice.